Differentiable Camera, Display, and Light
Cameras and displays have been developed to effectively capture and emit light. However, the design and control process of optics, hardware, and software for the cameras and displays has been developed in isolation, posing fundamental limits on acquiring, interacting, and analyzing light. In this course, we will study recent trends in differentiable camera and display designs to effectively capture and display light, the source of visual information. We will specifically focus on the following topics:
- Background on light properties
- High-dimensional imaging
- Differentiable camera and display
Time: October 10, afternoon (half day)
Organizer: Seung-Hwan Baek, POSTECH
Bio: Seung-Hwan Baek is an Assistant Professor at POSTECH Computer Graphics Lab, affiliated with the Department of Computer Science and Engineering and the Graduate School of AI.
His interests lie in computer graphics, computer vision, optics, imaging, display, and machine learning. In particular, he focuses on the joint design and optimization of optics, sensors, (neural) representations, and (neural) reconstructions for visual computing.
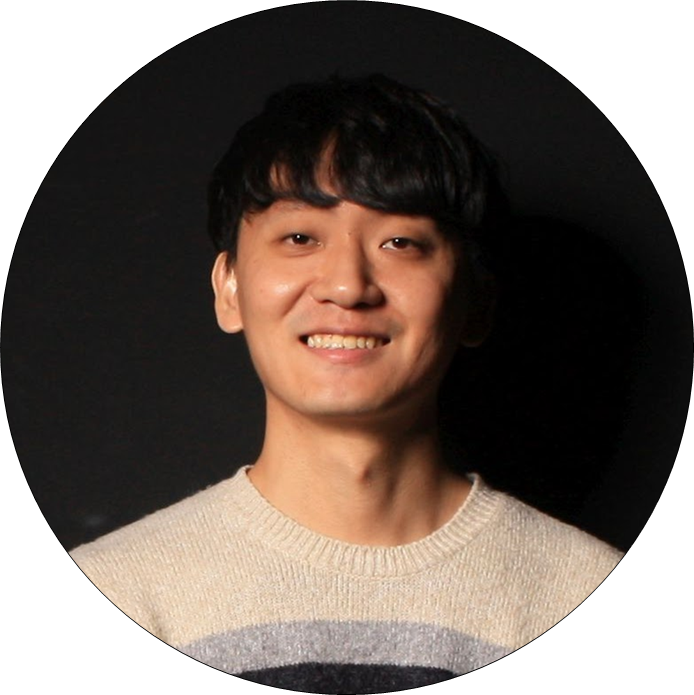
Slides: TBA
3D Generative Models: Recent Advances, Limitations, and Future Directions
Generative models for text and images have gained widespread popularity thanks to their remarkable advancements in producing highly realistic outputs. However, the challenge of generating 3D content remains largely unresolved, primarily due to the limited availability of large-scale data. Nevertheless, recent attempts to generate 3D content using 2D priors have shown surprising success, albeit with some instances of failure.
In the first part of the course, we will discuss recent advances in 3D generative models, with a focus on the fundamental components within the 3D generative model pipelines: NeRF, Diffusion Models, and Score Distillation Loss. Additionally, we will address the limitations of current pipelines and outline potential future research directions aimed at overcoming these challenges.
In this second part of the course, we will delve into the engineering considerations for 3D AI when applying these techniques in the industry. Specifically, we will discuss market experiences and explore future directions for providing 3D AI innovations as Software-as-a-Service (SaaS). The subtopics to be covered include ML-specific architectural considerations, cost factors, and user requirements.
Time: October 11, afternoon (half day)
Slides: Link
Organizers:
Minhyuk Sung, KAIST
Minhyuk Sung is an assistant professor in the School of Computing at KAIST, affiliated with the Graduate School of AI and the Metaverse Program. Before joining KAIST, he was a Research Scientist at Adobe Research. He received his Ph.D. from Stanford University under the supervision of Professor Leonidas J. Guibas. His research interests lie in vision, graphics, and machine learning, with a focus on 3D geometric data processing. His academic services include serving as a program committee member in SIGGRAPH Asia 2022 and 2023, Eurographics 2022 and 2024, and AAAI 2023 and 2024.
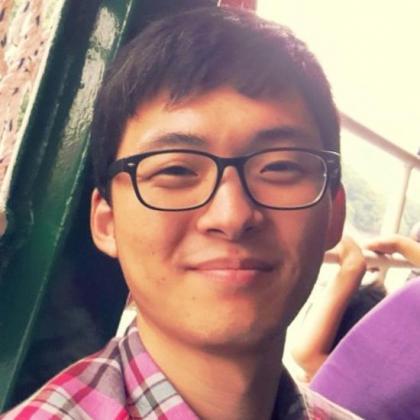
Kyungwon Yun, RECON Labs Inc.
Kyungwon Yun has been involved in numerous projects related to computer vision, graphics, and human-computer interaction, including areas such as pattern recognition, point cloud data, and RGBD camera application technology. He has also studied image processing and AI-related algorithms applied to microscopy and semiconductor inspection equipment. Recognizing the potential in neural rendering innovations of the 3D AI field, he joined RECON Labs Inc. as CTO and head of R&D. A passionate advocate for neural radiance fields and 3D Generative AIs, Dr. Yun now spearheads research to commercialize recent 3D AI research innovations into real-world products. Kyungwon earned his bachelor’s, master’s, and doctoral degrees in mechanical and aerospace engineering from Seoul National University, and a doctorate focusing on microscopic imaging using computer vision and AI.
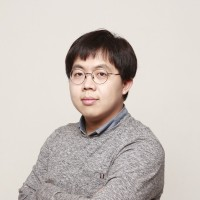